- Publications
- Conference paper
-
Achieving Participatory Smart Cities by Making Social Networks Safer
Achieving Participatory Smart Cities by Making Social Networks Safer
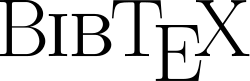
[u' @inproceedings{corcuera_achieving_2022, address = {Split}, title = {Achieving {Participatory} {Smart} {Cities} by {Making} {Social} {Networks} {Safer}}, isbn = {978-953-290-115-3}, abstract = {Cases of organised disinformation campaigns on Twitter, including those reported by the social network itself in its Transparency centre, continue unabated. The negative consequences of these attacks in processes of great importance to societies, such as electoral processes or vaccination campaigns, have sparked research into detecting this type of malicious user. State-of-the-art models for bot detection use numerous information collected from profiles, tweets, or network architecture to obtain competitive outcomes. On the other hand, these models allow for post-hoc detection of such users because they rely on fixed training datasets to classify users based on their previous activities. In contrast, we propose a proactive technique that uses user records to predict dangerous attacks before they occur as a measure to make social networks safer, fairer and less biased. For this purpose, our method uses a model that predicts malicious assaults by projecting users\u2019 embedding trajectories before completing their actions. We employed a Dynamic Directed Multigraph representation of temporal interactions between people in the Twittersphere for the experiments. By comparing them in the same data, our model outperforms state-of-the-art methods by 40.66\\% in F-score detecting malicious users preemptively. In addition, we propose a model selection study that evaluates the usefulness of several system components.}, language = {en}, booktitle = {Proceedings of the 7th {International} {Conference} on {Smart} and {Sustainable} {Technologies} ({Splitech} 2022)}, publisher = {FESB, University of Split}, author = {Corcuera, Ruben Sanchez and Zubiaga, Arkaitz and Almeida, Aitor}, month = apr, year = {2022}, keywords = {Foreseeing, Twitter, inception, machine learning, malicious users, social bots, social network analysis, social networks}, pages = {6}, } ']
Abstract