- Publications
- Journal article
-
Exploring LOD through metadata extraction and data-driven visualizations
Exploring LOD through metadata extraction and data-driven visualizations
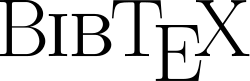
[u' @article{pena_exploring_2016, title = {Exploring {LOD} through metadata extraction and data-driven visualizations}, volume = {50}, issn = {0033-0337}, url = {http://www.emeraldinsight.com/doi/full/10.1108/PROG-12-2015-0079}, doi = {10.1108/PROG-12-2015-0079}, abstract = {Purpose \u2013 The purpose of this paper is to present a new approach toward automatically visualizing Linked Open Data (LOD) through metadata analysis.', u'Design/methodology/approach \u2013 By focussing on the data within a LOD dataset, the authors can infer its structure in a much better way than current approaches, generating more intuitive models to progress toward visual representations.', u'Findings \u2013 With no technical knowledge required, focussing on metadata properties from a semantically annotated dataset could lead to automatically generated charts that allow to understand the dataset in an exploratory manner. Through interactive visualizations, users can navigate LOD sources using a natural approach, in order to save time and resources when dealing with an unknown resource for the first time.', u'Research limitations/implications \u2013 This approach is suitable for available SPARQL endpoints and could be extended for resource description framework dumps loaded locally.', u'Originality/value \u2013 Most works dealing with LOD visualization are customized for a specific domain or dataset. This paper proposes a generic approach based on traditional data visualization and exploratory data analysis literature.}, number = {3}, urldate = {2016-06-10TZ}, journal = {Program}, author = {Pe\xf1a, Oscar and Aguilera, Unai and L\xf3pez-de-Ipi\xf1a, Diego}, month = jun, year = {2016}, note = {00000}, keywords = {Datatype Inference, Exploratory Data Analysis, JCR0.556, LOD Visualization, Linked Open Data, Metadata Extraction, q4, semantic web}, pages = {270--287} }']
Abstract