- Publications
- Journal article
-
Extending knowledge-driven activity models through data-driven learning techniques
Extending knowledge-driven activity models through data-driven learning techniques
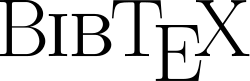
[u' @article{azkune_extending_2015, title = {Extending knowledge-driven activity models through data-driven learning techniques}, volume = {42}, issn = {0957-4174}, url = {http://www.sciencedirect.com/science/article/pii/S0957417414007623}, doi = {10.1016/j.eswa.2014.11.063}, abstract = {Knowledge-driven activity recognition is an emerging and promising research area which has already shown very interesting features and advantages. However, there are also some drawbacks, such as the usage of generic and static activity models. This paper presents an approach to using data-driven techniques to evolve knowledge-driven activity models with a user\u2019s behavioral data. The approach includes a novel clustering process where initial incomplete models developed through knowledge engineering are used to detect action clusters which represent activities and aggregate new actions. Based on those action clusters, a learning process is then designed to learn and model varying ways of performing activities in order to acquire complete and specialized activity models. The approach has been tested with real users\u2019 inputs, noisy sensors and demanding activity sequences. Initial results have shown that complete and specialized activity models are properly learned with success rates of 100\\% at the expense of learning some false positive models.}, number = {6}, urldate = {2015-01-20}, journal = {Expert Systems with Applications}, author = {Azkune, Gorka and Almeida, Aitor and L\xf3pez-de-Ipi\xf1a, Diego and Chen, Liming}, month = apr, year = {2015}, note = {00000}, keywords = {AI for health, Activity Annotation, Activity Recognition, Activity model, Artificial Intelligence, Data analysis, Knowledge-Driven, Q1, intelligent environments, jcr2.981, machine learning}, pages = {3115--3128}, } ']
Abstract