- Publications
- Conference paper
-
Influence Functions for Interpretable link prediction in Knowledge Graphs for Intelligent Environments
Influence Functions for Interpretable link prediction in Knowledge Graphs for Intelligent Environments
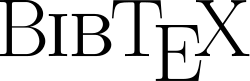
[u' @inproceedings{zulaika_zurimendi_influence_2022, title = {Influence {Functions} for {Interpretable} link prediction in {Knowledge} {Graphs} for {Intelligent} {Environments}}, isbn = {978-953-290-115-3}, abstract = {Knowledge graphs are large, graph-structured databases used in many use-case scenarios such as Intelligent Environments. Many Artificial Intelligent latent feature models are used to infer new facts in Knowledge Graphs. Despite their success, the lack of interpretability remains a challenge to overcome. This paper applies influence functions to obtain the most significant facts when predicting new knowledge and allows users to understand these models. However, Influence Functions do not scale well. We present an efficient method to scale up influence functions to large Knowledge Graphs to overcome such an issue. It drastically reduces the number of training samples when computing influences and uses fast curvature matrixvector products to linearize the computation steps required for the inverse Hessian. We conduct experiments on different sized Knowledge Graphs demonstrating the scalability of our approach and its effectiveness in measuring the most influential facts. Our method provides an intuitive understanding of link prediction behaviour in Knowledge Graphs and Intelligent Environments.}, language = {en}, booktitle = {Proceedings of the 7th {International} {Conference} on {Smart} and {Sustainable} {Technologies} ({Splitech} 2022)}, author = {Zulaika Zurimendi, Unai and Almeida, Aitor and Lopez-de-Ipina, Diego}, month = apr, year = {2022}, keywords = {Interpretability, inception, influence functions, knowledge graphs, link prediction, machine learning, representation learning}, pages = {7}, } ']
Abstract