- Publications
- Conference paper
-
Purity: a New Dimension for Measuring Data Centralization Quality
Purity: a New Dimension for Measuring Data Centralization Quality
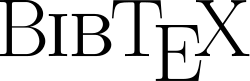
[u' @inproceedings{bonilla_purity_2024, address = {Oxford, UK}, title = {Purity: a {New} {Dimension} for {Measuring} {Data} {Centralization} {Quality}}, isbn = {979-8-4007-1725-3}, abstract = {Data has become an asset for companies, originating from various sources, such as IoT paradigms. It is crucial to safeguard its life cycle using suitable, scalable, and effective technologies, like those enabled by cloud computing models. However, in order to extract value from this data, complementary processes of collection, refinement, cleaning, or modeling, among many others, are required. Furthermore, organizations greatly vary in their methodologies and approaches to handling data, which further emphasizes the need for standardized techniques. In this regard, data management methodologies promote the adoption of the various dimensions of data quality in order to ensure the reliability of data across different systems and processes. The main contribution of this manuscript is the proposal of a new data quality dimension, coined purity, to measure the importance of the data in a processing pipeline topology. As a result, organizations can better guarantee the quality of their datasets in order to raise the success of data-driven endeavors within organizations. The proposed methodology is validated in an urban mobility use case.}, booktitle = {Proceedings of the 8th {International} {Conference} on {Cloud} and {Big} {Data} {Computing}}, publisher = {ACM}, author = {Bonilla, Lander and L\xf3pez Osa, Maria Jose and Diaz-de-Arcaya, Josu and Torre-Bastida, Ana and Almeida, Aitor}, month = aug, year = {2024}, keywords = {centralities, cloud computing, data quality, graph analysis, isi, networks, topology}, } ']
Abstract