- Publications
- Conference paper
-
Simplicity is Best: Addressing the Computational Cost of Machine Learning Classifiers in Constrained Edge Devices
Simplicity is Best: Addressing the Computational Cost of Machine Learning Classifiers in Constrained Edge Devices
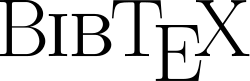
[u' @inproceedings{gomez-carmona_simplicity_2019, address = {Bilbao, Spain}, title = {Simplicity is {Best}: {Addressing} the {Computational} {Cost} of {Machine} {Learning} {Classifiers} in {Constrained} {Edge} {Devices}}, isbn = {978-1-4503-7207-7}, shorttitle = {Simplicity is {Best}}, url = {http://dl.acm.org/citation.cfm?doid=3365871.3365889}, doi = {10.1145/3365871.3365889}, abstract = {The potential of the Internet of Things (IoT) has traditionally grown upon the basis of its connectivity and communication capabilities, where low-power devices gather physical data and send them to remote high-performance nodes. However, the Edge Computing paradigm is changing the Cloud-based approach moving the processing and data computation towards the edge, getting the computation closer to the data source. As a consequence, extending intelligence to embedded platforms at the edge involves addressing differently the data processing and the computation techniques to overcome the constraints of the IoT devices. To contribute to this new challenge, we analyze the feasibility of deploying different supervised Machine Learning techniques applied to human activity recognition into two single-board computers, namely a Raspberry Pi 3B+ and a Raspberry Zero W. To that end, we present the classification example of a drinking activity monitoring system as a case study. The results show that an initial optimization process (i.e. selecting the most important features of the raw sensor data) is preeminent to provide a substantial improvement on the classification process with a minimal loss of performance and saving valuable computational cost. Thus, the presented approach seeks to stress the importance of understanding the initial data and studying the most relevant characteristics of the signal to overcome the limitations of the IoT devices and succeed in bringing embedded Machine Learning to the edge.}, language = {en}, urldate = {2019-11-11}, booktitle = {Proceedings of the 9th {International} {Conference} on the {Internet} of {Things} - {IoT} 2019}, publisher = {ACM Press}, author = {G\xf3mez-Carmona, Oihane and Casado-Mansilla, Diego and L\xf3pez-de-Ipi\xf1a, Diego and Garc\xeda-Zubia, Javier}, year = {2019}, keywords = {Edge Computing, Internet of Things, Smart Environments, machine learning}, pages = {1--8} }']
Abstract